Pushing Analytics Upstream: The Value of Processing Data at the Edge
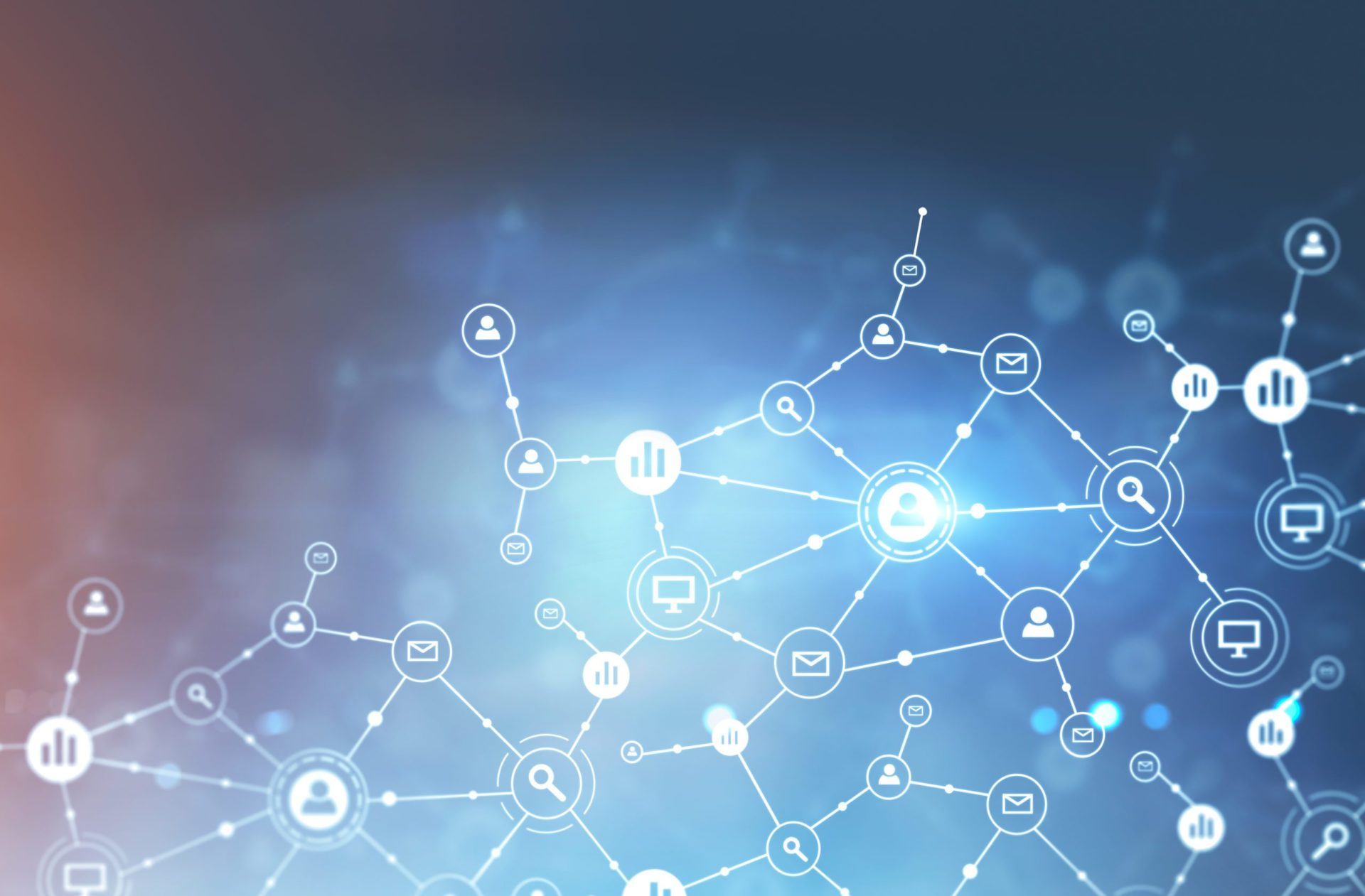
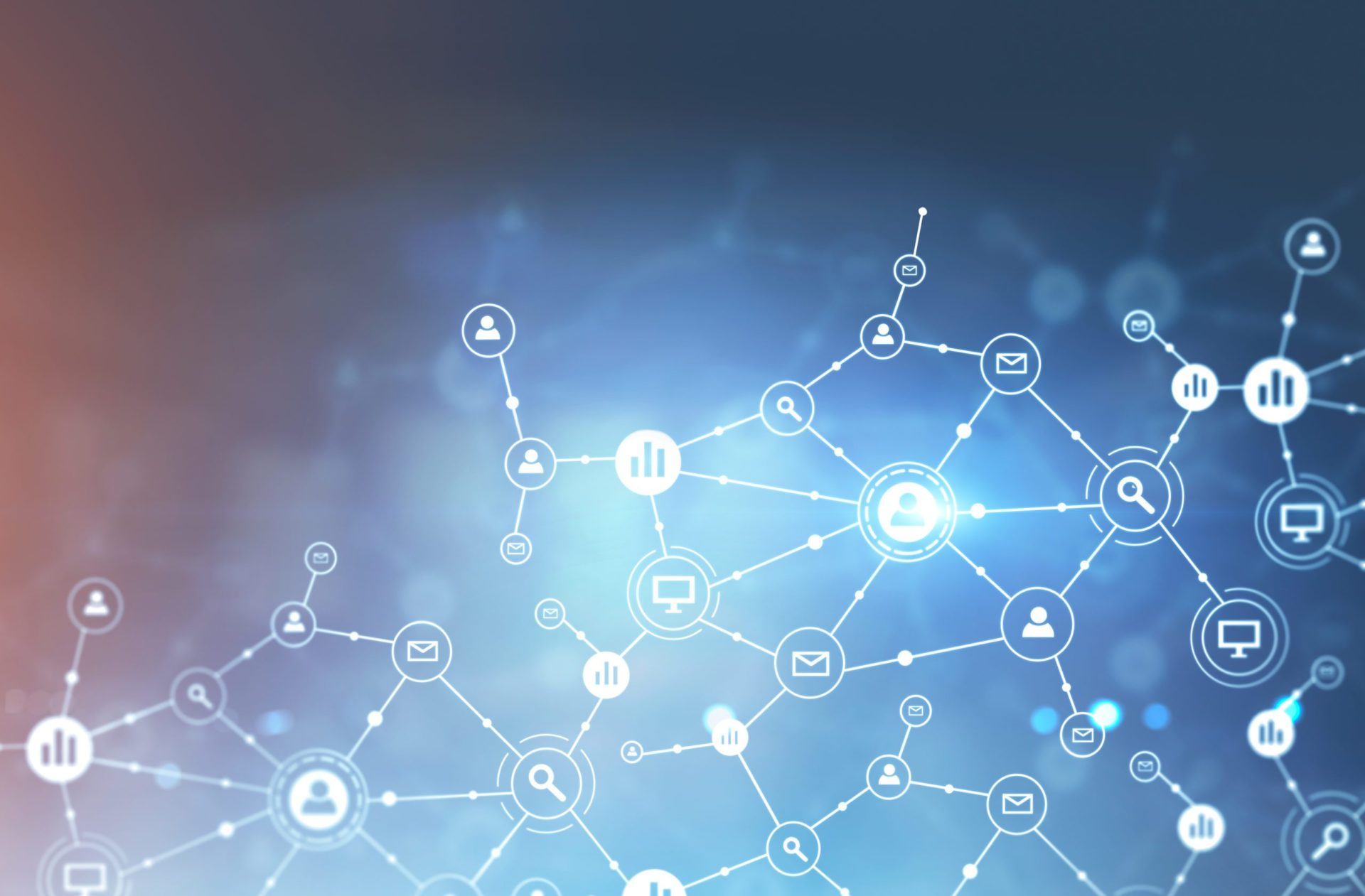
Ozan Unlu is the founder and CEO of Seattle-based Edge Delta, an edge observability platform. Previously, Unlu served as a senior solutions architect at Sumo Logic, a software development lead and program manager at Microsoft, and a data engineer at Boeing. He holds a B.S. in nanotechnology from the University of Washington.
For years, organizations have leveraged analytics with the goal of transforming data into insight and then action. Traditionally, many have relied on an approach known as “centralize and analyze,” where they pool all of their application, service, and system health data into a central repository for indexing and crunching.
In recent years, this approach has become increasingly problematic from a variety of perspectives, including the difficulty in keeping up with exploding data volumes and subsequently monitoring costs. As teams struggle to harness all of their data in order to optimize overall service health, they find themselves forced to make painful decisions regarding what data to analyze and what to neglect — a very risky proposition given the capricious nature of performance issues.
As a byproduct of this decision, teams often don’t have the data they need to anticipate or quickly resolve issues. This shows in the fact that despite technology advancements and the industry’s strong investment in resiliency, outages persist, with the number of outages lasting more than 24 hours increasing substantially.
Here, we’ll explore how a new approach to monitoring applications solves this problem. Rather than compressing and shipping massive data volumes to compute resources downstream, this new approach flips traditional monitoring on its head. Now, it is possible to push your compute to your datasets. Pushing data analytics upstream — or processing data at the edge — can help organizations overcome certain challenges and maximize the value of their data and analytics.
Analyzing all application and system health data at its source
Bringing compute resources geographically closer to users reduces latency and helps organizations deliver significantly better user performance as well as the ability to monitor new services without creating bottlenecks in downstream systems and on-premise data centers. Simply put, teams no longer need to predict upfront which datasets are valuable and worth analyzing in order to fix issues that can impact the customer’s/user’s experience.
Pushing analytics upstream to the edge can help organizations avoid such dilemmas by processing all application, service, and system health data at various points across the edge, simultaneously and broken down into bite-sized chunks. This allows organizations to effectively have an eye on all their data, without having to neglect even a single dataset.
Safeguarding and driving conversions
For transaction-heavy online services — e-commerce companies and travel booking sites, for example — highly performing applications and systems are the lifeblood of the business. When these applications go down — or even slow down, by as little as a few milliseconds — the result is a noticeable hit to conversion rates. According to statistics, the highest e-commerce conversion rates occur on sites with web page load times between 0-2 seconds, and with each additional second of load time, website conversion rates drop by an average of 4.42%. These statistics also note that a site that loads in one second has a conversion rate three times higher than a site that loads in five seconds.
In this context, mean-time-to-detect (MTTD) and mean-time-to-respond (MTTR) requirements are exceedingly slim, essentially zero. As discussed above, pushing analytics upstream enables teams to more proactively identify and address anomalies, while also intuitively pinpointing the exact location of growing hot spots or a particular infrastructure or application running on it. Teams can fix problems much faster, ideally before user performance is impacted in the first place — which is perhaps the most important step in safeguarding conversions.
But when it comes to actually increasing conversions, application and system health data are not the only type of data that can benefit from analytics being pushed further upstream. Today, nearly three out of every four dollars spent on online purchases is done so through a mobile device. A matter of nanoseconds can mean the difference between capitalizing on a site visitor’s ephemeral attention span — or not. When customer behavioral data is processed at the edge — thus avoiding long-distance communication flows back to the cloud — an organization can become much more agile and instantaneous in delivering highly personalized, high-velocity marketing that fuels conversions.
Keeping a lid on monitoring costs
The old “centralize and analyze” approach entailed routing all application and system health data to hot, searchable, and relatively expensive retention tiers. Many organizations experience sticker shock as they run up against, and in many cases, unknowingly exceed, data usage limits. One alternative is to purchase in advance more capacity than one may actually end up needing, but small businesses in particular can’t afford to be spending money on capacity they don’t ultimately use. Another drawback is the more data is in a repository, the longer the expected search time tends to be.
In the context of these challenges — and as edge processing grows — data stores need to follow suit. Gartner estimates that by 2025, 70% of organizations will switch their analytics approaches from “big” to “small and wide,” and a key enabling factor is that the edge offers tremendous flexibility and creates space for more real-time data analysis across larger volumes of data. When analytics are pushed upstream, organizations process their data then and there, right at the source. From there, teams can move their data to a more low-cost storage option in the cloud, where it remains searchable.
Whether driven by compliance requirements, the desire to mine historical data for further analysis, or something else — there are going to be occasions where teams do need access to all their data. In these cases, it will be there, readily and easily available to anyone who needs it, without exhausting or exceeding budgets in the process.
Conclusion
As data volumes grow exponentially, processing data at the edge becomes the most feasible way to cost-effectively and comprehensively leverage an organization’s rich data. Now, teams can realize the potential to analyze all of their data to ensure high performance, uptime, and strong user experiences.