The Future of AI Is Tiny
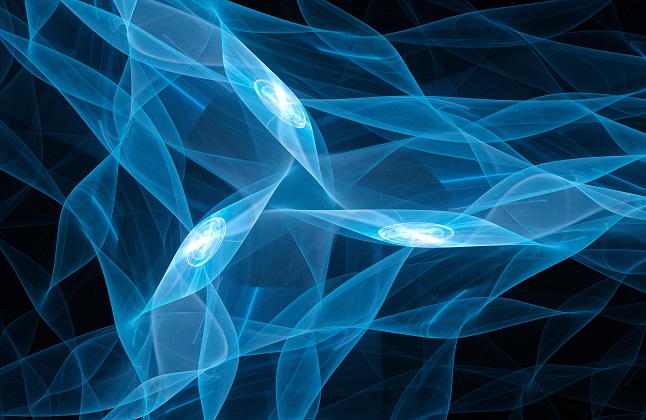
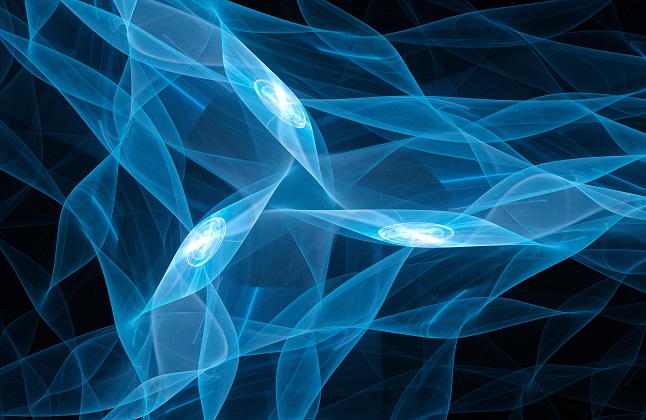
Over the years, technology has progressively miniaturized in dimension and costs, which has led to mass adoption. From bulky, energy-consuming computers, we now have smartwatches packing the same punch at a microscopic fraction of size and power. While these innovations have led to compact, portable, and efficient solutions, they have also spawned technological advances like the budding field of nanotechnology. AI is next to be embedded into our lives as it will be deeply intertwined into all spheres of human activity.
Need for Tiny AI
Research conducted by the University of Massachusetts Amherst
reveals that training one single algorithm might generate five times the lifetime carbon dioxide emissions of an average automobile. Some language processing algorithms work with a colossal data set of 340 million data parameters and for training it, the costs and energy expenditure and emissions levels are astoundingly high. Such models deliver highly accurate results but have extreme energy and computational demands.
Ceaseless pursuit of the highest accuracy possible in AI models has sometimes created a myopia, where costs, operational efficiency, latency have been overlooked, all coming at the cost of an expanded carbon footprint. There also are some industrial use cases where running AI is simply not feasible.
How Can AI Become Tiny?
Tiny AI is a breakthrough technology, which attempts to compress and shrink existing AI models to reduce their complexity, making them efficient and faster, reducing costs, and energy requirements. Through careful data selection, compact model architectures, and various techniques like knowledge distillation, quantization and pruning, developers can optimize and shrink the original AI models to consume significantly less processing power and data, without comprising the accuracy.
This results in a massive reduction in complexity and load, making it possible to run locally on smart edge devices and not send it to the cloud every time. At the edge, power and processing requirements are much lower, cutting down on costs and bandwidth requirements. Reduced workload for training and inferencing on cloud servers also increases execution speed.
Tiny AI researchers from Huawei and Huazhong University of Science and Technology were successful at building Tiny BERT based on the NLP model BERT, which was 7.5 times smaller than the parent model but 9.4 times faster with significantly less energy expenditure.
Although Tiny AI also can offer sustainability benefits, to achieve mass adoption solely based on smaller AI models and edge processing capabilities would be difficult. Federated learning, battery-less IoT sensors, and decentralized network coverage are three external advances that will further expand adoption of this technology and enhance its sustainability.
Business Benefits
Tiny AI, in principle, already exists in our lives in smartphones, where AI-based assistants are doing detection and inferencing, without requiring internet access and heavy computational power. Organizations have initiated applications in which electricity providers use tiny AI to prevent wildfires, by detecting potential areas of occurrence and taking preventive action.
Tiny AI could also deliver the following:
- Sustainability goals and environmental credentials: AI’s carbon footprint is more than that of the airline industry, and with the rising number of IOT devices, this problem will reach alarming proportions. By making more light-weight models, we can achieve unparalleled efficiency gains and increase adoption, without comprising on sustainability goals.
- Smart and intelligent platforms: Tiny AI could fundamentally alter our interaction with many devices by bringing powerful deep learning at an affordable cost to create context-aware consumer devices. The list of services and technologies, like computer vision, voice assistants, text and speech processing, image processing in cameras, autonomous driving, manufacturing, financial platforms, connected healthcare, Industry 4.0 and intelligent logistics, is endless. The reduction in costs due to lesser computational power will generate a wave of responsive devices and the demand for them will grow exponentially causing them to scale rapidly.
- Integral for highly regulated and privacy conscious segments: Potential low to no data requirements for cloud storage will be a boon for industries in which data collection and storage is a cause for concern. In edge devices, the models are personalized to the edge environment and critical personal data never leaves the device. Tiny AI will appeal to this segment much more as it solves the problem by reducing the data infrastructure and bolstering security.
- Mobility, robotics, and manufacturing: Driven by the three pillars of cost-effectiveness, energy efficiency and ultra-fast processing, these industries will be naturally suited for implementations like quality checks, predictive maintenance, alerting, anomaly detection, enabling a vast workforce of digital workers to assume several aspects.
Conclusion
When implementing AI, it is important to be aware that environmental costs don’t outweigh both the perceived and real benefits of the technology. To future-proof the AI ecosystem, we will need greater focus on sustainability measures, cost reductions in training, and implementation.
Corporate leadership must now start embedding sustainable practices in their use of AI. Visionary executives will appreciate the value presented by tiny AI on the edge — as it saves money and energy while improving data privacy and security.